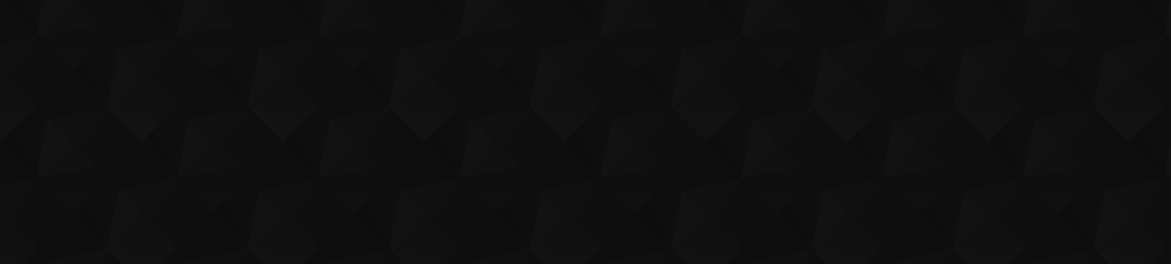
- 615
- 2 239 028
Tübingen Machine Learning
Germany
Приєднався 31 бер 2020
This is the youtube channel of the machine learning groups at the University of Tübingen
Theorie II - 16 - Turing-Vollständigkeit und - Äquivalenz
Diese Vorlesung stammt aus dem Kurs
"Theoretische Informatik II: Formale Sprachen, Berechenbarkeit, Komplexität"
von Prof. Dr. Philipp Hennig, gehalten im Sommersemester 2024 an der Universität Tübingen.
Die Veranstaltung wird auf Deutsch gehalten und richtet sich an BSc-Studierende der Informatik im 3. und 4. Fachsemester.
Auf unserem Kanal finden Sie auch die Partnerversion dieser Vorlesung von Prof. Dr. Ulrike von Luxburg, aus dem (Corona) Sommer 2021: ua-cam.com/play/PL05umP7R6ij24rRWOuvbg4ucfO6ncG2Dj.html&feature=shared
"Theoretische Informatik II: Formale Sprachen, Berechenbarkeit, Komplexität"
von Prof. Dr. Philipp Hennig, gehalten im Sommersemester 2024 an der Universität Tübingen.
Die Veranstaltung wird auf Deutsch gehalten und richtet sich an BSc-Studierende der Informatik im 3. und 4. Fachsemester.
Auf unserem Kanal finden Sie auch die Partnerversion dieser Vorlesung von Prof. Dr. Ulrike von Luxburg, aus dem (Corona) Sommer 2021: ua-cam.com/play/PL05umP7R6ij24rRWOuvbg4ucfO6ncG2Dj.html&feature=shared
Переглядів: 205
Відео
Theorie II - 12 - Nicht Erkennbar / Nicht Entscheidbar
Переглядів 8014 годин тому
Diese Vorlesung stammt aus dem Kurs "Theoretische Informatik II: Formale Sprachen, Berechenbarkeit, Komplexität" von Prof. Dr. Philipp Hennig, gehalten im Sommersemester 2024 an der Universität Tübingen. Die Veranstaltung wird auf Deutsch gehalten und richtet sich an BSc-Studierende der Informatik im 3. und 4. Fachsemester. Auf unserem Kanal finden Sie auch die Partnerversion dieser Vorlesung v...
Theorie II - 15 - Das Post'sche Korrespondenzproblem
Переглядів 4414 годин тому
Diese Vorlesung stammt aus dem Kurs "Theoretische Informatik II: Formale Sprachen, Berechenbarkeit, Komplexität" von Prof. Dr. Philipp Hennig, gehalten im Sommersemester 2024 an der Universität Tübingen. Die Veranstaltung wird auf Deutsch gehalten und richtet sich an BSc-Studierende der Informatik im 3. und 4. Fachsemester. Auf unserem Kanal finden Sie auch die Partnerversion dieser Vorlesung v...
Theorie II - 14 - Satz von Rice
Переглядів 6314 годин тому
Diese Vorlesung stammt aus dem Kurs "Theoretische Informatik II: Formale Sprachen, Berechenbarkeit, Komplexität" von Prof. Dr. Philipp Hennig, gehalten im Sommersemester 2024 an der Universität Tübingen. Die Veranstaltung wird auf Deutsch gehalten und richtet sich an BSc-Studierende der Informatik im 3. und 4. Fachsemester. Auf unserem Kanal finden Sie auch die Partnerversion dieser Vorlesung v...
Theorie II - 13 - Reduktionen
Переглядів 7814 годин тому
Diese Vorlesung stammt aus dem Kurs "Theoretische Informatik II: Formale Sprachen, Berechenbarkeit, Komplexität" von Prof. Dr. Philipp Hennig, gehalten im Sommersemester 2024 an der Universität Tübingen. Die Veranstaltung wird auf Deutsch gehalten und richtet sich an BSc-Studierende der Informatik im 3. und 4. Fachsemester. Auf unserem Kanal finden Sie auch die Partnerversion dieser Vorlesung v...
Theorie II - 11 - Abzählbarkeit
Переглядів 5114 годин тому
Diese Vorlesung stammt aus dem Kurs "Theoretische Informatik II: Formale Sprachen, Berechenbarkeit, Komplexität" von Prof. Dr. Philipp Hennig, gehalten im Sommersemester 2024 an der Universität Tübingen. Die Veranstaltung wird auf Deutsch gehalten und richtet sich an BSc-Studierende der Informatik im 3. und 4. Fachsemester. Auf unserem Kanal finden Sie auch die Partnerversion dieser Vorlesung v...
Theorie II - 09 - Turingmaschinen
Переглядів 3214 годин тому
Diese Vorlesung stammt aus dem Kurs "Theoretische Informatik II: Formale Sprachen, Berechenbarkeit, Komplexität" von Prof. Dr. Philipp Hennig, gehalten im Sommersemester 2024 an der Universität Tübingen. Die Veranstaltung wird auf Deutsch gehalten und richtet sich an BSc-Studierende der Informatik im 3. und 4. Fachsemester. Auf unserem Kanal finden Sie auch die Partnerversion dieser Vorlesung v...
Theorie II - 08 - Deterministische Kontextfreie Grammatiken
Переглядів 3614 годин тому
Diese Vorlesung stammt aus dem Kurs "Theoretische Informatik II: Formale Sprachen, Berechenbarkeit, Komplexität" von Prof. Dr. Philipp Hennig, gehalten im Sommersemester 2024 an der Universität Tübingen. Die Veranstaltung wird auf Deutsch gehalten und richtet sich an BSc-Studierende der Informatik im 3. und 4. Fachsemester. Auf unserem Kanal finden Sie auch die Partnerversion dieser Vorlesung v...
Theorie II - 07 - Kellerautomaten
Переглядів 2414 годин тому
Diese Vorlesung stammt aus dem Kurs "Theoretische Informatik II: Formale Sprachen, Berechenbarkeit, Komplexität" von Prof. Dr. Philipp Hennig, gehalten im Sommersemester 2024 an der Universität Tübingen. Die Veranstaltung wird auf Deutsch gehalten und richtet sich an BSc-Studierende der Informatik im 3. und 4. Fachsemester. Auf unserem Kanal finden Sie auch die Partnerversion dieser Vorlesung v...
Theorie II - 06 - Kontextfreie Grammatiken
Переглядів 4014 годин тому
Diese Vorlesung stammt aus dem Kurs "Theoretische Informatik II: Formale Sprachen, Berechenbarkeit, Komplexität" von Prof. Dr. Philipp Hennig, gehalten im Sommersemester 2024 an der Universität Tübingen. Die Veranstaltung wird auf Deutsch gehalten und richtet sich an BSc-Studierende der Informatik im 3. und 4. Fachsemester. Auf unserem Kanal finden Sie auch die Partnerversion dieser Vorlesung v...
Theorie II - 05 - Das Pumping-Lemma
Переглядів 2814 годин тому
Diese Vorlesung stammt aus dem Kurs "Theoretische Informatik II: Formale Sprachen, Berechenbarkeit, Komplexität" von Prof. Dr. Philipp Hennig, gehalten im Sommersemester 2024 an der Universität Tübingen. Die Veranstaltung wird auf Deutsch gehalten und richtet sich an BSc-Studierende der Informatik im 3. und 4. Fachsemester. Auf unserem Kanal finden Sie auch die Partnerversion dieser Vorlesung v...
Theorie II - 04 - Reguläre Ausdrücke
Переглядів 2914 годин тому
Diese Vorlesung stammt aus dem Kurs "Theoretische Informatik II: Formale Sprachen, Berechenbarkeit, Komplexität" von Prof. Dr. Philipp Hennig, gehalten im Sommersemester 2024 an der Universität Tübingen. Die Veranstaltung wird auf Deutsch gehalten und richtet sich an BSc-Studierende der Informatik im 3. und 4. Fachsemester. Auf unserem Kanal finden Sie auch die Partnerversion dieser Vorlesung v...
Theorie II - 03 - Nichtdeterministische Endliche Automaten
Переглядів 8714 годин тому
Diese Vorlesung stammt aus dem Kurs "Theoretische Informatik II: Formale Sprachen, Berechenbarkeit, Komplexität" von Prof. Dr. Philipp Hennig, gehalten im Sommersemester 2024 an der Universität Tübingen. Die Veranstaltung wird auf Deutsch gehalten und richtet sich an BSc-Studierende der Informatik im 3. und 4. Fachsemester. Auf unserem Kanal finden Sie auch die Partnerversion dieser Vorlesung v...
Theorie II - 02 - Deterministische Endliche Automaten
Переглядів 20414 годин тому
Diese Vorlesung stammt aus dem Kurs "Theoretische Informatik II: Formale Sprachen, Berechenbarkeit, Komplexität" von Prof. Dr. Philipp Hennig, gehalten im Sommersemester 2024 an der Universität Tübingen. Die Veranstaltung wird auf Deutsch gehalten und richtet sich an BSc-Studierende der Informatik im 3. und 4. Fachsemester. Auf unserem Kanal finden Sie auch die Partnerversion dieser Vorlesung v...
Theorie II - 01 - Formale Sprachen
Переглядів 25714 годин тому
Diese Vorlesung stammt aus dem Kurs "Theoretische Informatik II: Formale Sprachen, Berechenbarkeit, Komplexität" von Prof. Dr. Philipp Hennig, gehalten im Sommersemester 2024 an der Universität Tübingen. Die Veranstaltung wird auf Deutsch gehalten und richtet sich an BSc-Studierende der Informatik im 3. und 4. Fachsemester. Auf unserem Kanal finden Sie auch die Partnerversion dieser Vorlesung v...
Trustworthy ML - Lecture 12 - Conclusion (Exam, Final topics, Research at STAI)
Переглядів 6374 місяці тому
Trustworthy ML - Lecture 12 - Conclusion (Exam, Final topics, Research at STAI)
Trustworthy ML - Lecture 11 - Uncertainty (Aleatoric uncertainty, Factorisation)
Переглядів 6165 місяців тому
Trustworthy ML - Lecture 11 - Uncertainty (Aleatoric uncertainty, Factorisation)
Trustworthy ML - Lecture 10 - Uncertainty (Definitions & evaluation, Epistemic uncertainty)
Переглядів 8425 місяців тому
Trustworthy ML - Lecture 10 - Uncertainty (Definitions & evaluation, Epistemic uncertainty)
Virtual Humans -- Lecture 07.2 Fitting SMPL to IMU Learning
Переглядів 5876 місяців тому
Virtual Humans Lecture 07.2 Fitting SMPL to IMU Learning
Virtual Humans -- Lecture 09.1 Neural Implicits and Point Based Clothing Models PART2
Переглядів 2166 місяців тому
Virtual Humans Lecture 09.1 Neural Implicits and Point Based Clothing Models PART2
Virtual Humans -- Lecture 09.1 Neural Implicits and Point Based Clothing Models PART3 and PART4
Переглядів 3746 місяців тому
Virtual Humans Lecture 09.1 Neural Implicits and Point Based Clothing Models PART3 and PART4
Virtual Humans -- Lecture 09.1 Neural Implicits and Point Based Clothing Models PART2
Переглядів 5496 місяців тому
Virtual Humans Lecture 09.1 Neural Implicits and Point Based Clothing Models PART2
Virtual Humans -- Lecture 09.1 Neural Implicits and Point Based Clothing Models PART1
Переглядів 4866 місяців тому
Virtual Humans Lecture 09.1 Neural Implicits and Point Based Clothing Models PART1
Trustworthy ML - Lecture 9 - Uncertainty (Definitions & evaluation)
Переглядів 7566 місяців тому
Trustworthy ML - Lecture 9 - Uncertainty (Definitions & evaluation)
Trustworthy ML - Lecture 8 - Explainability (Training data attribution)
Переглядів 6126 місяців тому
Trustworthy ML - Lecture 8 - Explainability (Training data attribution)
Trustworthy ML - Lecture 7 - Explainability (Feature attribution, Training data attribution)
Переглядів 6796 місяців тому
Trustworthy ML - Lecture 7 - Explainability (Feature attribution, Training data attribution)
Trustworthy ML - Lecture 6 - Explainability (Feature attribution)
Переглядів 6967 місяців тому
Trustworthy ML - Lecture 6 - Explainability (Feature attribution)
Trustworthy ML - Lecture 5 - OOD Generalisation (Adversarial defenses), Explainability (Definitions)
Переглядів 6267 місяців тому
Trustworthy ML - Lecture 5 - OOD Generalisation (Adversarial defenses), Explainability (Definitions)
Trustworthy ML - Lecture 4 - OOD Generalisation (Adversarial attacks)
Переглядів 6817 місяців тому
Trustworthy ML - Lecture 4 - OOD Generalisation (Adversarial attacks)
Trustworthy ML - Lecture 3 - OOD Generalisation (Cue selection)
Переглядів 9667 місяців тому
Trustworthy ML - Lecture 3 - OOD Generalisation (Cue selection)
Is it correct that the way we define x on slide 10 the order matters? Otherwise p(x|f) would not be a probability distribution. I think this is quite inconsistent with the way x was used before: Not as some vector in {0,1}^n but as the number of glasses-wearing people -> Would be more consistent to use a binomial coefficient as normalization constant.
Danke fürs hochladen!
Tübingen ML has some of the best educational UA-cam thumbnails. Usually very clean.
Thanks.
good video
Best video so far on ANOVA
1:23:40
next lecture please
The best video on this topic I have found so far by a large margin. Excellent work!
Hi , Please provide code and excercise of this very nice code.
Can we get course website
You are the best teacher in the world thanks
It was mentioned earlier that the product of two GP is another GP only if it is over the same set of variables (x), and that it is some else if it is over two different set of variables (say x and y). Does not this apply to the prediction step at 1:17:11 (from 2nd to 3rd line)?
Great video!
Having watched quite a lot regression videos I can say confidently this is something which sums up and condenses each and every thing for a beginner to grasp linear regression smoothly(see what I did there?). Thank you so much for making this public!
27:20 Really impressive, especially the pronunciation of Kolmogorov's name.
have you tried with silver nano wire networks ?
awesome
sehr gut
Which book do you guys follow?
can we have the homeward or exercises of this course?
Maike hat das toll gemacht!
in 36:40 sum( f_i(\theta) P_i), P has dimension 3N and f(\theta) has 207? how this multiplication is possible?
Answer is also on the SMPL paper with a better notation
Awesome class. But the notation in slide 11 is a bit confusing, what is the advantage of having a unity vector and rotation angle on \omega_j? And, is there a typo on slide 11 should \omega² be (\omega_j)²?
okay, answer is in the SMPL paper 😀
where can i get the code ?
this is the first lecture that went right over my head.....something to look forward to
Exceptional Course!!
This is the BEST course in the world on machine learning!! Others start with linear regression being a prob with parameter w. Prof Philipp start with how probability is a measure on sets. there is no other course in the world that explains this much
I second that, btw do you have the codes for the lecture ?
Exceptional!!!!
At 1:04:13 , ua-cam.com/video/36GT2zI8lVA/v-deo.html
47:00
It is hard to understand CNN.
Gràcies per fer-ho obert!
Thanks a lot for greay content
Thank you Mr. Hennig. You are an outstanding lecturer in a complex field. A wonderful integrative development of ideas. Enables us to grasp the ensemble of concepts. Thank you.
This is masterpiece. The narrative. Coherence, unity. A real beautiful piece of work.
I think this was the most important lecture of the course, pure gold! There is a big Dilema between making better models in the sense of decreasing error in particular datasets and understanding what is being done. I mean, from the research point of view, it does not seem very interesting in just producing better results if it doesnt come with something that is understandable. Actually, as the lecturer pointed out, Deep Learning research is flooded with that type of thing. GPUs are making people lazy, in the sense of just applying heuristics everywhere, wheres the beauty in that methodology?
can we get the slides, if permissible?
Absolutely amazing video course. Especially after looking at other sources I notice how valuable this is. Every video achieves to combine the intuition and math in a concise was. I recommend the videos to anyone who wants to learn about ML.
Point to the surface optimization is really amazing!
M(\vec{\theta}, \vec{\beta}; \mathbf{T}, \mathcal{S}, \mathcal{P}, \mathcal{W}, \mathcal{J})) 😍
At 44:00 why does the professor say this works for any non zero probability set? I feel P(A ∪ Φ) = P(A) + P(Φ) and thus P(A) = P(A) + P(Φ) and so P(Φ) = 0 for any P(A)
1019
Thanks for these amazing lectures..
amazing lecture. Please post more videos.
Thank you sir for the wonderful explanation
I am tired.
Is there a signal processing course published publicly? He mentioned one in the video but I couldn't find it.
This is probably the most complicated course I've watched.
That's just how we roll. :)
@ Could you provide the codes in the course?
I am afraid.
Me too